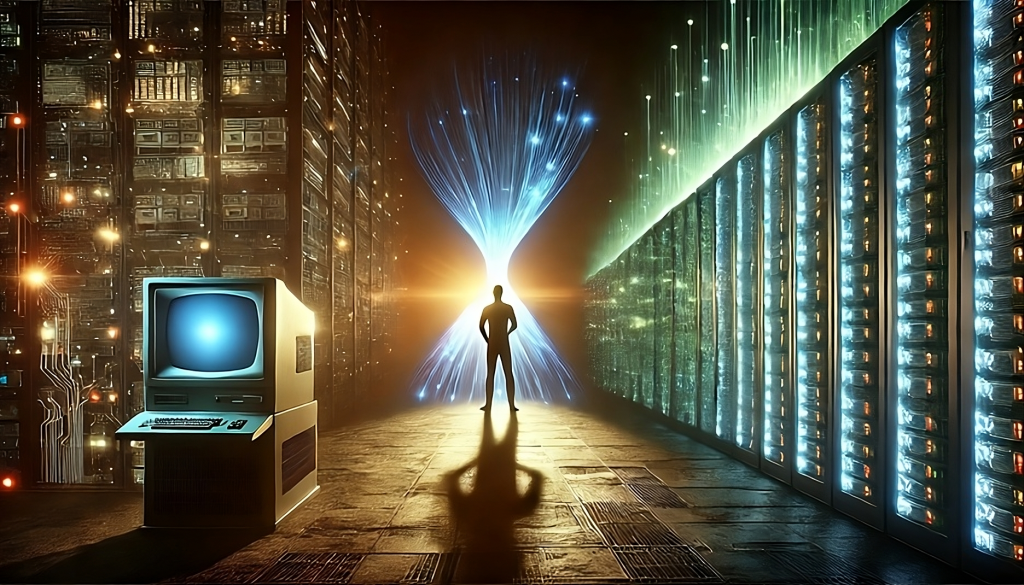
Join our daily and weekly newsletters for newest updates and exclusive content to cover the industry. Learn more
In recent Nvidia GTC conference, the company reveals what is described first single-rack system in an exchlop, or a set of festivities (flops-point) per second. This blow is based on the latest GB200 NVL72 system, which includes new Blackwell processing units in NVIDIA (GPU). A standard computer rack is about 6 feet long, a little more than 3 feet depth and less than 2 feet wide.
Shrink an exinoflop: from forward to Blackwell
A couple things about the announcement strikes me. First, the first release of the world with competent computer was set several years ago, at 2022, at the Oak Ridge National Laboratory. For comparison, the “Frontier” supercomputer built by the HPE and operated by AMD GPUs and CPU, origin of 74 racks on servers. The new NVIVIA system achieves nearly 73x more density of performance in just three years, corresponding to a tripling of performance each year. This improvement reflects the rare development of computing density, Emerger Efficiency and architecture design.
Second, it is necessary to say that while the two systems hit Milestone on Exascale, they are built for different challenges, one that is optimized for speed. EXINOFLOP fixed by NVIDIA is based on mathematical waters – specifically 4-bit and 8-bit AI’s 8-bit functions including the functions of accuracy of accuracy. In contrast, ExinOflop rating for Frontier has achieved the use of 64-bit double-fecision mathematics, the gold standard for similiated scientific simulations if accuracy is important.
We come a long way (very quickly)
This level of development seems almost incredible, especially when I remember the state-of-of-ths when I started my career in the computing industry. My first professional job as a programmer of December 1090 except CPU performance, the machine connected with the Learned Ray Tube (CRT) appeared through hardwired cables. There are no graphical capabilities, lighting only text on a dark background. And of course, no internet. The remote users are connected to telephone lines using modems running speeds up to 1,200 bits per second.

500 billion times more compute
While comparing MIPs of flops gives a general sense of development, it is important to remember that these standards measure different computers. MIPs show the integer processing speed, which is useful for general computer objective, especially with business applications. Flops measure the fluating-point performance that is important for scientific scientific and heavy figures in modern AI, such as matrix models and running machines (ML) models.
Although not a direct comparison, the more measure of the difference between MIPs then and flops now gives a strong description of strong growth. Using it as a moderate heuristic to measure the work made, the new NVIDIA system is estimated to be 500 billion over the machine more powerful than the dechi machine. That kind of jump reflects the exponential growth of computing power in a professional career and produces the question: what can this progress in 40 years, what does the next 5 come to do?
NVIDIA, for its part, offering some signs. In GTC, the company contributes to a roadmap predicting the next generation system based on “Vera Rubin” ultrach architecture this year, reaching an Ultra Rack in Ultra Rack in Ultra.
Just as acquaintance is recovery. Achieving this level of performance in a rack means less physical space per unit of work, fewer use of powerful use of these systems.
Does the AI really need everyone calculated loudly?
While such performance profits are impressive, the AI industry currently drives a fundamental question: what is necessary in a computation power and how much it costs? The race to build a large number of new AI AI data is driven by the growing demands of exascale exascale computation.
The more ambitious effort is $ 500 billion stargate project, thinking of 20 data centers across the US, half a half-square-square. A wave of other hyperscale projects is initiated or in the world’s planning periods, as companies and countries scramble to ensure that the infrastructure to support AI workloads at AI tomorrow.
Some analysts are now concerned that we can enlarge AI data capacity. The anxiety intensified after the release of R1, a model of rationalization from the Chinese Dreereek requires less to save more than most of its peers. Microsoft describes leases with multiple data providers, sparking considering it is more likely to change expectations for future AI infrastructure.
However, The registry proposed That this pullback may have additional do with some planned AI data centers without enough powerful ability to support gen ai systems. Today, AI models push the limits of what can be supported by current infrastructure. Technology Review of MIT reported that it can cause many Chinese data centers are struggling and failed, built in details that are unimportant for the present need, let the next need today.
AI demands immorsefully many flops
Modforming models have performed most of their runtime work through a process known to advertise. These models are the power of some of the most advanced and applications of strong resources today, including deep research assistants and the developmental waves of the ageo systems.
While Dereseek-R1 originally found the thought industry that the future AI may need less The power of computing, Nvidia CEO Jensen Huang pushes back. speaking In CNBC, he treats this understanding: “This is exactly the opposite of the conclusion that everyone is.” He added that reasoning AI uses 100x more computers than not arguing ai.
While AI continues from the autonomous agent rational agents and more, the need for computation is likely to rise again. The next blows can come not only in language or sight, but in the coordination of AI AG Agen, each person can by the type of jumping incompatibility we have just won.
Seemed right to cue, just notified of the openii $ 40 billion new fundsthe largest private tech funds gathered. The company said to a Blog post that the fund “allows us to push the boundaries of AI research yet, scaling our compute infrastructure and delivering more powerful tools for 500 million people using chatgpts.”
Why is so much capital flowing to AI? The reasons from the National Security competition. Even if a certain factor means, as shown in a McKinsey TITLE: “AI can increase corporate income by $ 4.4 trillion a year.”
What’s next to come? This is the prophecy of anyone
In their core, information systems regarding abstracting complexity, even in an emergency vehicle rate system previously written in Forol, or Modern AI Systems facilitating drug discovery. The goal is always the same: to make the most of the world’s understanding.
Now, with a strong AI that begins to show, we cross a door. For the first time, we can have the power to compute and intelligence to solve problems that may not be reached by man.
New York Timesist Kevin Roose recently got this opportunity: “Every week, I met the engineers and businessmen who told me that the change, changing the change in the world, around the corner.” And no one can count on the explosions that come every week.
In the past few days, we saw GPT-4o in OpenII almost perfect images From the text, Google’s release is what can be the most advanced Model that argues Despite Gemini 2.5 Pro and Runway revealing a video model with shooting character and scene conjunction, an item of Bentureat BAD Fast majority of video generators in AI so far.
What is next next. We don’t know if AI is a breakthrough or breakthrough, if it helps to solve Fusion energy or load new biological risks. But with frequent flops that come online in the next five years, one thing is certain: the innovation comes quickly – and in force. Also, as such flops scale, so we need our conversations about responsibility, regulation and control.
Gary Grossman is EVP in technology practice in Edelman and global lead in Edelman Ai Center in Korma.
Source link